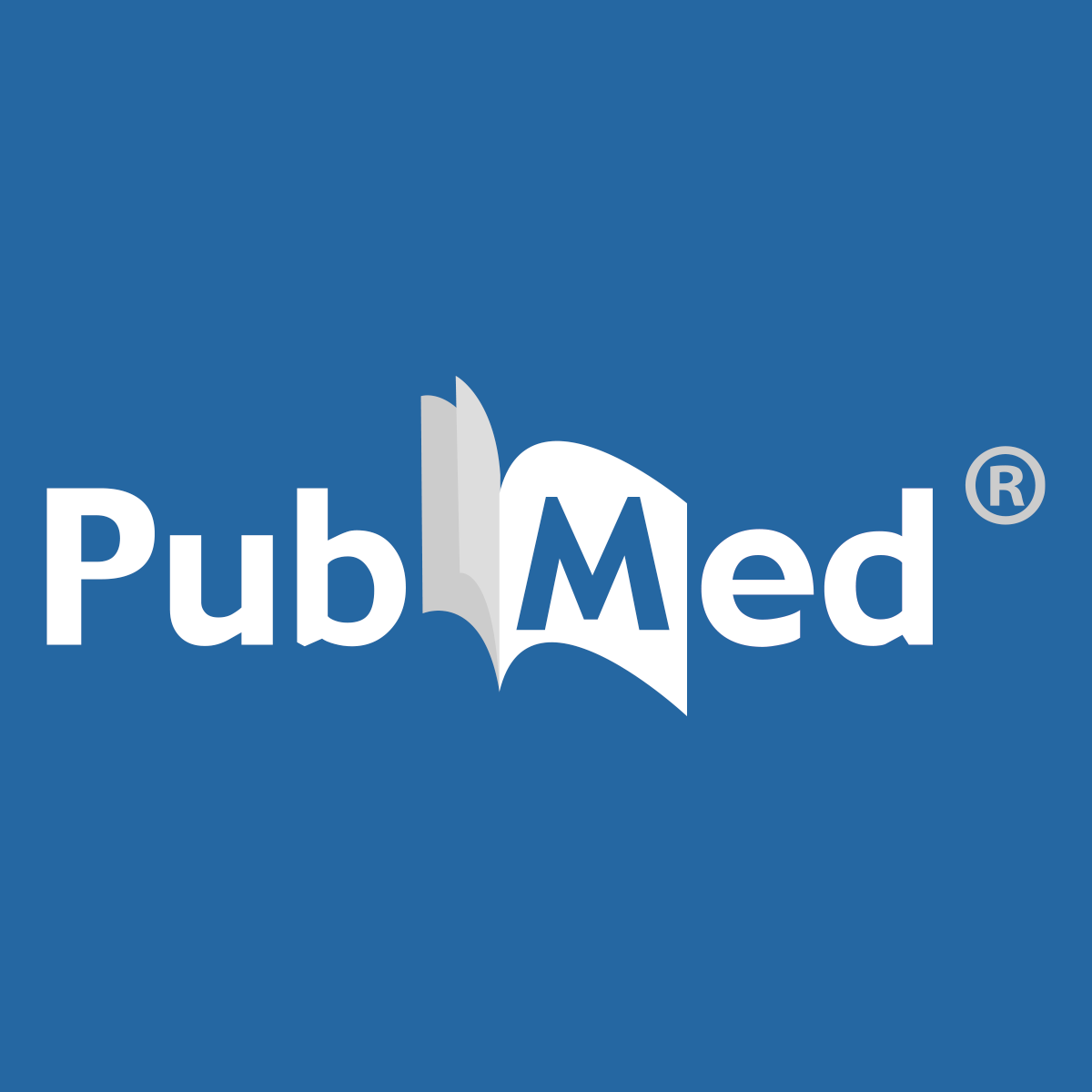
J Environ Radioact. 2022 Feb;242:106787. doi: 10.1016/j.jenvrad.2021.106787. Epub 2021 Dec 4.
ABSTRACT
During a nuclear accident, estimating the source terms using environmental measurements is vital for emergency decision-making. In this study, we propose a forecasting model based on a temporal convolutional network to estimate the release rates of seven radionuclides (Kr-88, Te-132, I-131, Xe-133, Cs-137, Ba-140, and Ce-144) based on off-site sequential gamma dose rates and meteo rological monitoring data. To determine the best structure of the neural network, Bayesian optimization and hyperband (BOHB) was used on the hyperparameters of the model to reduce the testing loss. Additionally, a gradient boosting regression model was used to predict missing gamma dose rates to ensure the model offers a relatively reliable estimate under certain circumstances. The international radiological assessment system (InterRAS) was used to generate datasets for model training and testing. The results showed that the optimal hyperparameters selected by BOHB can reduce the valid loss of the model to 0.0153, and the mean absolute percentage error of prediction for the seven radionuclides was below 12%, three of which (Kr-88, Te-132, Cs-137) reached 8% at 10 h. When the first and second time-steps of the data were missing, the mean absolute percentage error of the prediction for all radionuclides was less than 30% after using a gradient boosting regression.
PMID:34875444 | DOI:10.1016/j.jenvrad.2021.106787
No comments:
Post a Comment